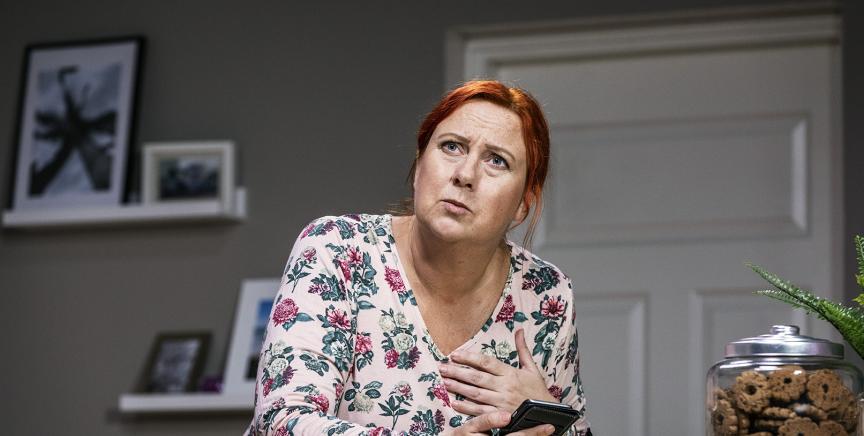
Atrial fibrillation – clinical significance
Atrial fibrillation (AFib) is the most commonly occurring type of arrhythmia, affecting nearly 1 percent of the population under the age of 60 years.13-16 It is estimated that 2.2 million Americans have AFib and 160,000 new cases are diagnosed each year.1 AFib is especially prevalent in elderly adults because of AFib risk associated with advanced age.2 AFib has become responsible for significant morbidity and mortality, and is a significant contributor to healthcare expense.3 Hospitals spend 17 percent more on patients with AFib than on non-AFib patients.4 Hospital admissions for AFib are related to hemodynamic compromise, decompensation of preexisting heart failure and treatment of complications from thromboembolism and stroke, as well as for evaluation and treatment for AFib itself.2, 5 AFib increases the risk for stroke by three to five times. The National Stroke Association reported that in 1998, 11.4 percent (80,000/700,000) of strokes were a complication of AFib.6 AFib is also a common condition resulting from heart surgery, and develops in 11 percent to 40 percent of patients after coronary artery bypass grafting and in more than 50 percent of patients after valvular surgery.7 This condition is the leading cause of prolonged hospital stays and extended recovery.6, 7 Clinical presentation of AFib is variable and can range from complete absence of symptoms to syncope, shortness of breath, palpitations, heart failure or hemodynamic collapse. Symptoms are related to loss of atrial systole and to irregular and rapid R-R intervals. The presentation of symptoms depends on the presence or absence of underlying structural heart disease, patient age, AV nodal conduction properties and the use of drug therapy.4
Mechanisms of AFib
The mechanisms of AFib are not completely understood. However, it is believed that multiple wavelets resulting from atrial reentry may cause the continuous impulses during AFib.8 Changes to the atrial muscle, such as atrial dilatation and fibrosis, are important factors in the occurrence and maintenance of AFib.9 These changes are often a result of rheumatic and ischemic heart disease, hypertension and congestive heart failure. In addition, AFib itself can lead to atrial dilatation and can perpetuate itself.10 Recent studies show that prolonged episodes of AFib can lead to persistent shortening of atrial refractoriness or electrical remodeling.11, 12
Implications for identifying AFib
Recent studies suggest that early conversion of AFib back to sinus may help prevent electrical remodeling and reduce the risk of chronic AFib.12 Identification of AFib is therefore critical to treating AFib and monitoring potential complications as a result of AFib. The duration of the AFib episode, the patient‘s symptoms and heart rate are collectively important in determining a treatment and/or preventative intervention for the risk of thromboembolism and other serious complications.
AFib identification during patient monitoring
The GE Healthcare AFib detection algorithm attempts to address the need for identifying AFib by providing an alarm and a time-based trend of the state of atrial fibrillation within a patient. The alarm is designed to identify the onset of AFib within two minutes of presentation to the algorithm. The time-based trend tracks the state of AFib (AFib present vs. AFib not present) over time and is presented on the heart rate trend. The AFib detection algorithm is incorporated into the GE EK-Pro arrhythmia analysis algorithm, which is used in GE monitoring products. It is a combination of a predictive model (Hidden Markov Model) used in GE Holter Systems and supporting logic for the patient monitoring environment . The supporting logic is shown in the process map of Figure 1. It consists of atrial analysis by detection of P waves, contextual analysis by identification of similarities and/or patterns in the R-R intervals, and lastly, a state evaluation that applies a setof rules for determining the current state of AFib.
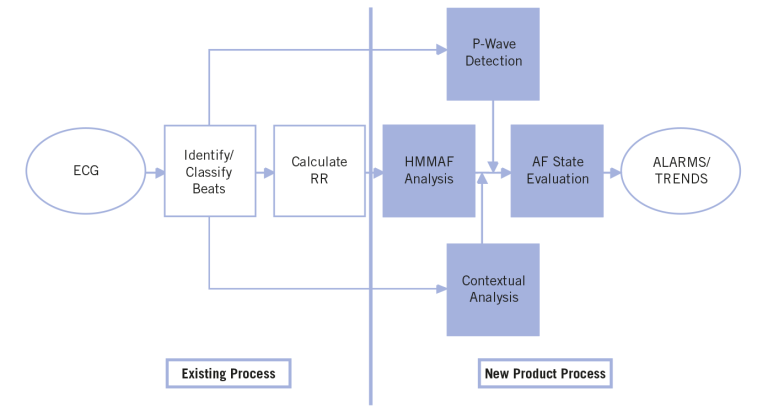
Hidden Markov Model
The Hidden Markov Model algorithm for AFib detection determines the probability that a given R-R interval sequence is part of an AFib rhythm. It analyzes sequences of three R-R intervals between normal morphology beats, scores thesequence, filters the output and determines if the rhythm isAFib or non-AFib. The lookup table was trained using a set ofrecords from the MIT/BIH arrhythmia database and is hard-coded into the algorithm.
P-wave detection
P-wave detection is performed to identify atrial activity within the patient‘s normal rhythm. The GE EK-Pro arrhythmia analysis algorithm maintains a template representing the morphology of the patient‘s dominant beat type. This template is used for identifying P waves. The detector searches back from the QRS to find wave onset, peak and offset. The deflections of the wave between the onset and peak and the offset and peak are compared to a threshold to determine if a P wave present. This is demonstrated in Figure 2.
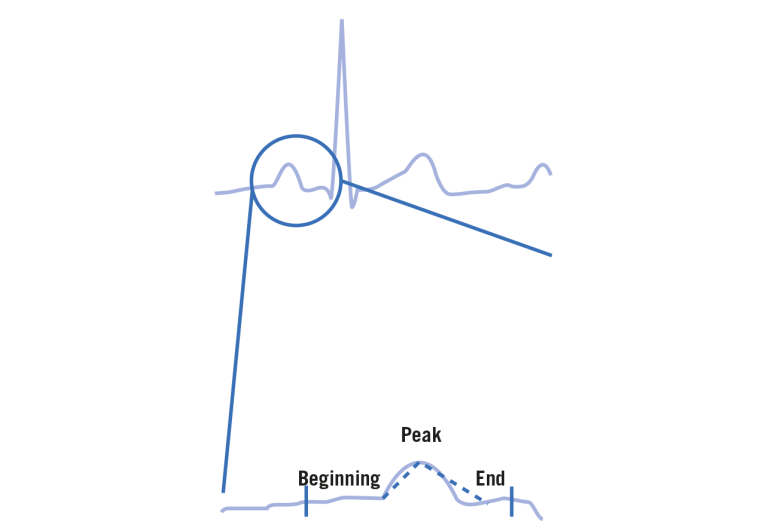
Time-based trend to identify AFib
This algorithm only attempts to identify when AFib is present. No additional processing occurs in this algorithm to identify other non-AFib rhythms when AFib is not present. In addition, there is the capability to display a time-based trend of the AFib state information. Because of the many AFib treatment options, many clinicians want to know patient status over time. This is addressed with a trended variable that can be output to the host system, providing an overall look at a patient‘s condition.
Figure 3 is an example of the heart rate trend and the AFib trend. The state of AFib over time is plotted below the heart rate and can be recognized by the solid line.
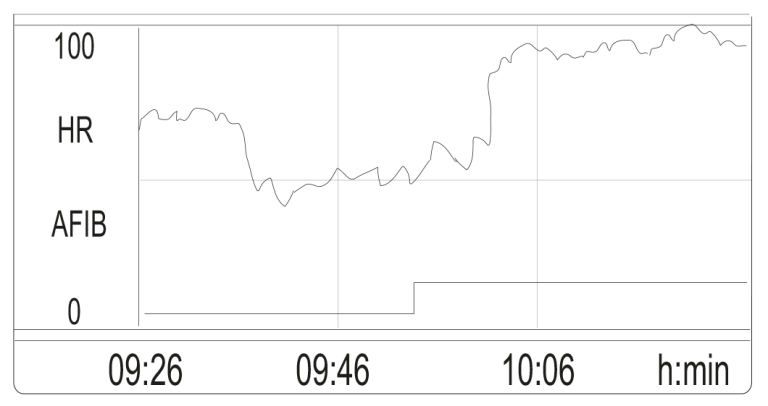
Algorithm performance
During the product development process the GE algorithm was tested for positive predictive accuracy and sensitivity. Positive predictive accuracy was tested on real-time clinical data. A CDT-LAN telemetry cabinet (hereafter called the “test tower”) was installed at a local hospital for engineering test and development. The test tower is physically isolated from any hospital monitoring networks and information systems. The only information collected by the test tower are signals from the telemetry transmitters worn by patients in the care areas. No confidential patient information is available from the test tower. ECG data from up to six patients at one time was supplied for approximately 24 hours to the algorithm and reviewed to assess performance statistics. Each day, six new patients were chosen at random to be monitored for AFib. No patient types were excluded. ECG history events and trend data were reviewed and printed for all patients processed by the test tower. The history strips (10 seconds of data at the time of the alarm) were used to determine if, at the time of the alarm, the patient was in AFib and evaluated for correctness and clinical acceptance. All strips were validated by a clinical cardiologist. Results are shown in Table 1. One hundred and eight patients were monitored, equaling 2,024 hours of time. Nineteen of the 108 patients had at least one episode of AFib, four of which had more than one episode. Episodes ranged anywhere between three minutes and 24 hours. Because testing was concerned with the determination of the validity of the alarms, true and false negatives were not considered and a measure of sensitivity was not available.
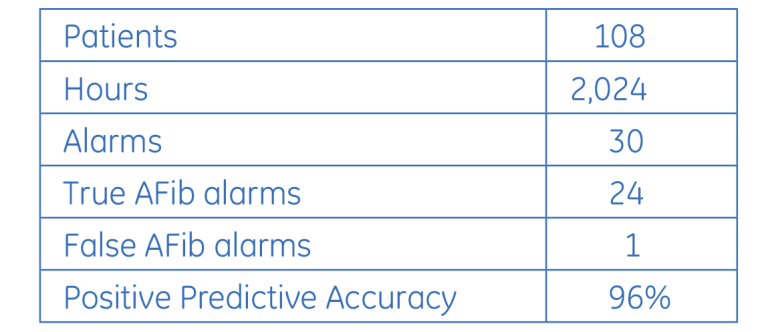
In addition to the real-time testing, off-line testing of a database was used. The database consisted of 33 records, of which 18 records had an episode of AFib. These records were collected using the same system as in the real-time tests, but were logged to a full-disclosure record system and reviewed on a PC. The records were chosen specifically to represent patients with AFib and other irregular rhythms, and are not representative of the population as a whole. The results are shown in Table 2. Each of the 18 records with AFib had only one episode of AFib, ranging from 18 minutes to almost 10 hours.
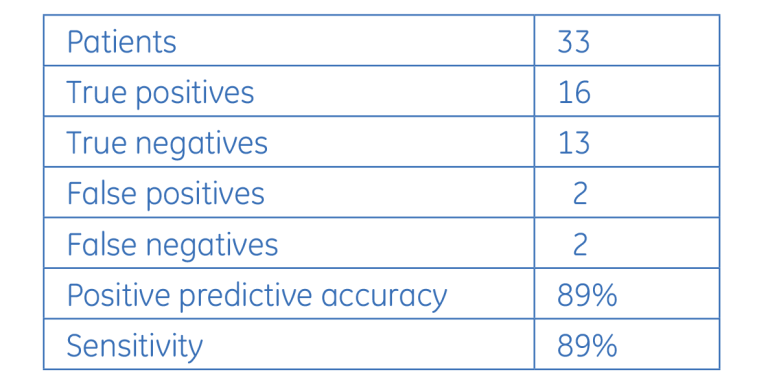
Conclusion
Atrial fibrillation is a widespread affliction with implications for increased risk of more serious health conditions. Monitoring patients to identify AFib can aid in developing an effective management plan that may prevent the condition from becoming chronic, therefore reducing potential health risks, complications and healthcare costs.
References
- Baily D. et al. Hospitalizations for arrhythmias in the United States: Importance of atrial fibrillation, (abstr.) J Am Coll Cardiol. 19, 41A (1991).
- Benjamin E. J. et al. Independent risk factors for atrial fibrillation in a population-based cohort. JAMA 271, 840-44 (1994).
- Kerr C.R. Guest editorial — Atrial Fibrillation: The Next Frontier. PACE 17, 1203-07, (1994).
- Sra J. Atrial fibrillation: epidemiology, mechanisms, and management. Current Problems in Cardiodology 25, 405-524 (2000).
- Wolfe P. A. et al. Atrial fibrillation: A major contributor to stroke in the elderly. Arch Intern Med. 147, 1561-64, (1987).
- Stroke risk factors and their impact. National Stroke Association, (1999).
- Reed III, G. L. et al. Stroke following coronary-artery bypass surgery. New Engl J Med. 319, 1246-50, (1988).
- Waldo A. L. Mechanisms of atrial fibrillation, atrial flutter and ectopic atrial tachycardia — A brief review 75 (suppl III), III-37-III-40, (1987).
- Janse M. J. Why does atrial fibrillation occur? Eur Heart J. 18 (suppl C), C12-C18, (1997).
- Wiejffels M. C. E. F. et al. Atrial fibrillation begets atrial fibrillation: A study in awake chronically instrumented goats. Circulation 92, 1954-68 (1995).
- Daoud E. G. et al. Effect of atrial fibrillation on atrial refractoriness in humans. Circulation 94, 1600-06, (1996).
- Franz M. R. et al. Electrical remodeling of the human atrium: Similar effects in patients with chronic atrial fibrillation and atrial flutter. J Am Coll Cardiol. 30, 1785-92 (1997).
- Flegel K.M. et al. Risk of stroke in non-rheumatic atrial fibrillation. Erratum in: Lancet 1 878, 526-9 (1987)
- Wolf P. A. et al. Atrial fibrillation as an independent risk factor for stroke: the Framingham study. Stroke 22, 983-8 (1991).
- Furberg C. D. et al. Prevalence of atrial fibrillation in elderly subjects (the Cardiovascular Health Study). American Journal of Cardiology 74, 236-41 (1994)
- Fuster C. D. et al. ACC/AHA/ESC guidelines for the management of patients with atrial fibrillation. A report of the American College of Cardiology/American Heart Association Task Force on Practice Guidelines and the European Society Cardiology Committee for Practice Guidelines and Policy Conferences (Committee to develop guidelines for the management of patients with atrial fibrillation) developed in collaboration with the North American Society of Pacing and Electrophysiology. European Heart Journal 22, 1852-923 (2001).
Additional resources
For white papers, guides and other instructive materials about GE Healthcare’s clinical measurements, technologies and applications, please visit http://clinicalview.gehealthcare.com/