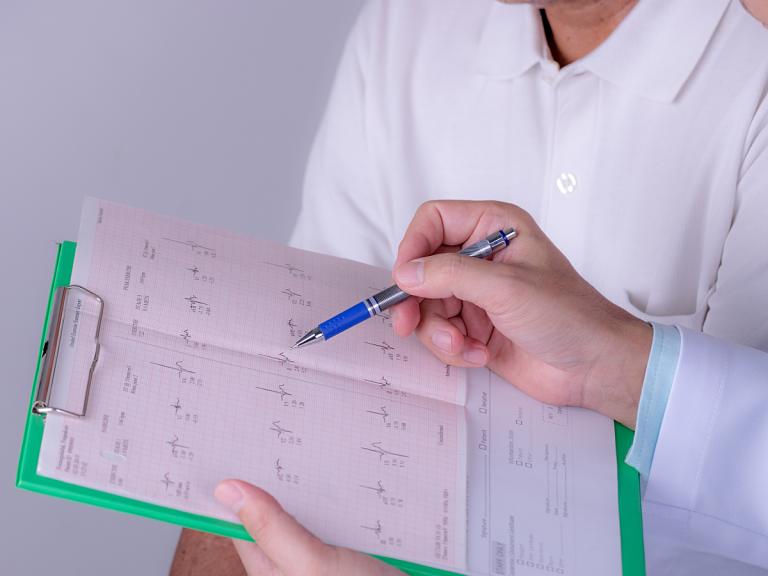
In the context of interpreting exercise ECGs, the ST segment remains an important variable.1 However, other variables may affect the test's sensitivity and specificity, causing false positives and ultimately interfering with the diagnostic or screening process.
According to previous research examining more than 24,000 patients, ECG stress testing for coronary artery disease has a sensitivity of 68% and specificity of 77%.2 As research continues, these numbers may be improved using other measurements, like heart-rate corrected analysis of ST segment variables (ST/HR) hysteresis, and newer technologies, including convoluted neural networks (CNN). Although these may supplement the physician's interpretation of exercise ECG results, a thorough understanding of sensitivity and specificity helps provide accurate diagnoses.
Reviewing Sensitivity and Specificity
Many clinicians struggle with the concepts of sensitivity and specificity, as they essentially approach diagnostic problems in reverse.1 At its most basic, sensitivity is the ability of any test or screening exam to detect true positives. Put another way, any test's sensitivity is its ability to correctly identify all individuals with a specific medical condition.3 In contrast, specificity refers to a test's ability to detect true negatives; in other words, specificity is a test's ability to correctly identify individuals who do not have a specific medical condition.3
Both sensitivity and specificity can be expressed as a mathematical equation featuring four variables, a through d, which are assigned to represent:
- True positive (a)
- False positive (b)
- False negative (c)
- True negative (d)
The mathematical equations for sensitivity and specificity present as:3
- Sensitivity = [a/(a+c)] × 100
- Specificity = [d/(b+d)] × 100
However, both variables are open to misinterpretation when compared to reference standards and other measurements. In the case of sensitivity, reference standards typically do not account for people who do not have the suspected medical condition. Additionally, these equations may not account for other complications, such as deficiencies in reference standards or in the test itself.3
The Importance of ST/HR Hysteresis
ST/HR has been demonstrated to increase sensitivity and specificity in both male and female patients.1 This measurement takes the exercise and recovery phases of an exercise ECG into account, improving the diagnostic and prognostic ability of exercise ECG.4 ST/HR hysteresis may be considered more valuable from a diagnostic perspective: it integrates the difference in ST segment depression spanning the exercise and recovery phases over the patient's heart rate for up to three minutes during recovery.1
How Neural Networks Can Aid Diagnosis
In recent years, CNNs have found increasing applications in various aspects of the medical field, and cardiology is no exception. CNNs are a type of deep neural network, so called because of its many layers compared to other types of neural networks.5 Clinicians typically use CNNs to analyze visual data, including exercise ECG results. In various research studies, CNNs demonstrate greater diagnostic accuracy compared to interpretation by experts.5
In one study consisting of more than 4,000 ECGs, an investigational 11-layer CNN showed 98.33% sensitivity and 98.35% specificity.6 Other studies suggest that CNNs can accurately interpret large ECG data sets to achieve better sensitivity and specificity values than clinical cardiologists.7
When it comes to accurately interpreting sensitivity and specificity, advancing technologies will continue to improve values in various diagnostic and screening modalities, including exercise ECG. Clinicians should be aware of the potential advantages of such systems while also maintaining a thorough understanding of the variables.
Resources:
- GE Healthcare: Physician's Guide to GE Stress Systems, 2051167-002 ENG.
- Matta M, Harb SC, Cremer P, et al. Stress testing and noninvasive coronary imaging: what's the best test for my patient? Cleveland Clinic Journal of Medicine. 2021;88(9):502-515. https://www.ccjm.org/content/88/9/502.
- Trevethan R. Sensitivity, specificity, and predictive values: foundations, pliabilities, and pitfalls in research and practice. Front Public Health. 2017;5. https://www.frontiersin.org/articles/10.3389/fpubh.2017.00307/full.
- Carlén A, Nylander E, Åström Aneq M, et al. Physiological Reports. 2019;7(2):e13968. https://doi.org/10.14814/phy2.13968
- Health IT Analytics. Types of deep learning & their uses in healthcare: deep learning is a growing trend in healthcare artificial intelligence, but what are the use cases for the various types of deep learning? https://healthitanalytics.com/features/types-of-deep-learning-their-uses-in-healthcare. Accessed January 3, 2023.
- Avanzato R, Beritelli F. Automatic ECG diagnosis using convolutional neural network. Electronics. 2020;9(6):951. https://www.mdpi.com/2079-9292/9/6/951/htm
- Hughes JW, Olgin JE, Avram R, et al. Performance of a convolutional neural network and explainability technique for 12-lead electrocardiogram interpretation. The Journal of the American Medical Association Cardiology. 2021;6(11):1285-1295. https://jamanetwork.com/journals/jamacardiology/fullarticle/2782549